Atlas of the Possible, Probable and Improbable Airports
Rethinking Airport Spatial Analysis and Design using GAN and Aerial Imagery
Abstract
Airports require long term planning, balancing estimations of future demand against available airfield land and site constraints. This is becoming more critical with climate change and the transition to sustainable aviation fuelling infrastructure. This paper demonstrates a novel procedure using Satellite Imagery and Generative Learning to aid in the comparative analysis and early-stage airfield design. Our workflow uses a GAN trained on 2000 images of airports transforming them into a high-dimensional latent space capturing the typologies' large-scale features. Using a process of projection and dimensional-reduction methods we can locate real-world airport images in the generative latent space and vice-versa. With this capability we can perform comparative “neighbour” analysis at scale based on spatial similarity of features like airfield configuration, and surrounding context. Using this low-dimensional 3D 'airport designs space' with meaningful markers provided by existing airports allows for 'what if' modelling, such as visualizing an airport on a site without one, modifying an existing airport towards another target airport, or exploring changes in terrain, such as due to climate change or urban development. We present this method a new way to undertake case study, site identification and analysis, as well as undertake speculative design powered by typology informed ML generation, which can be applied to any typologies which could use aerial images to categorize them.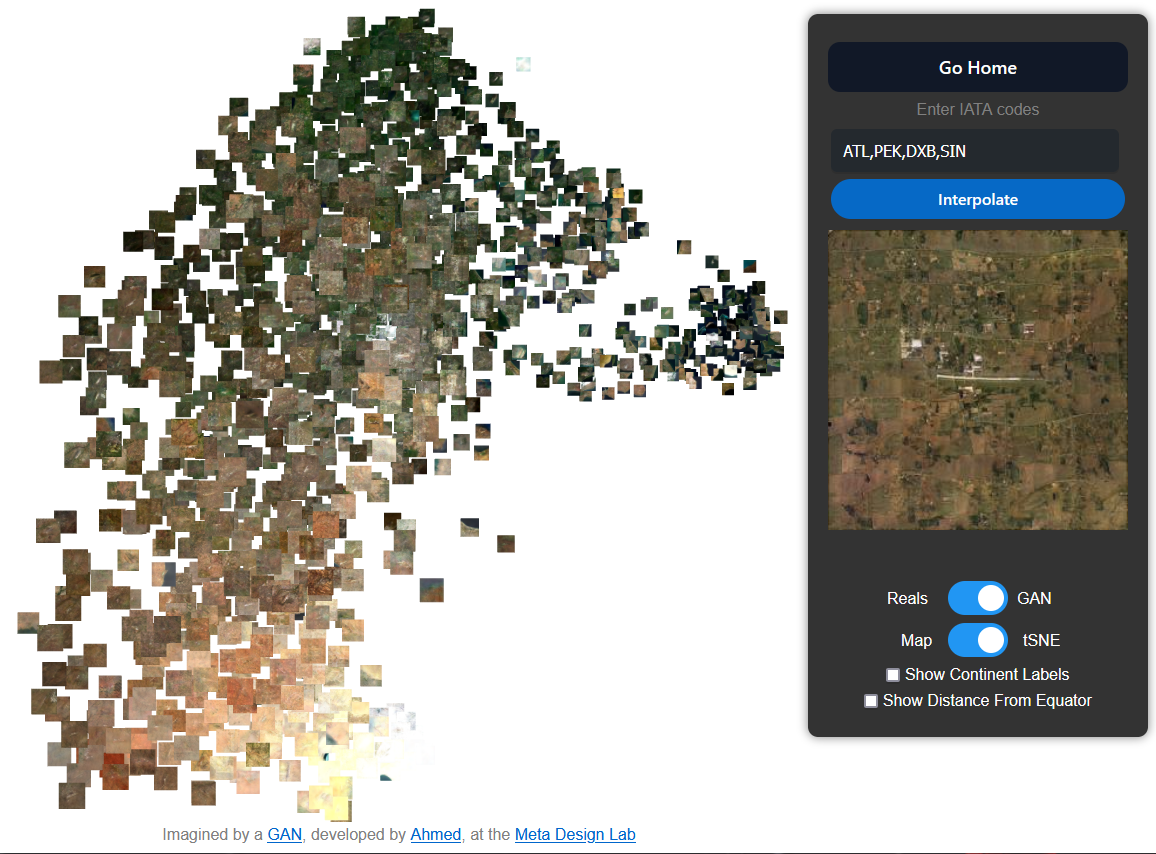
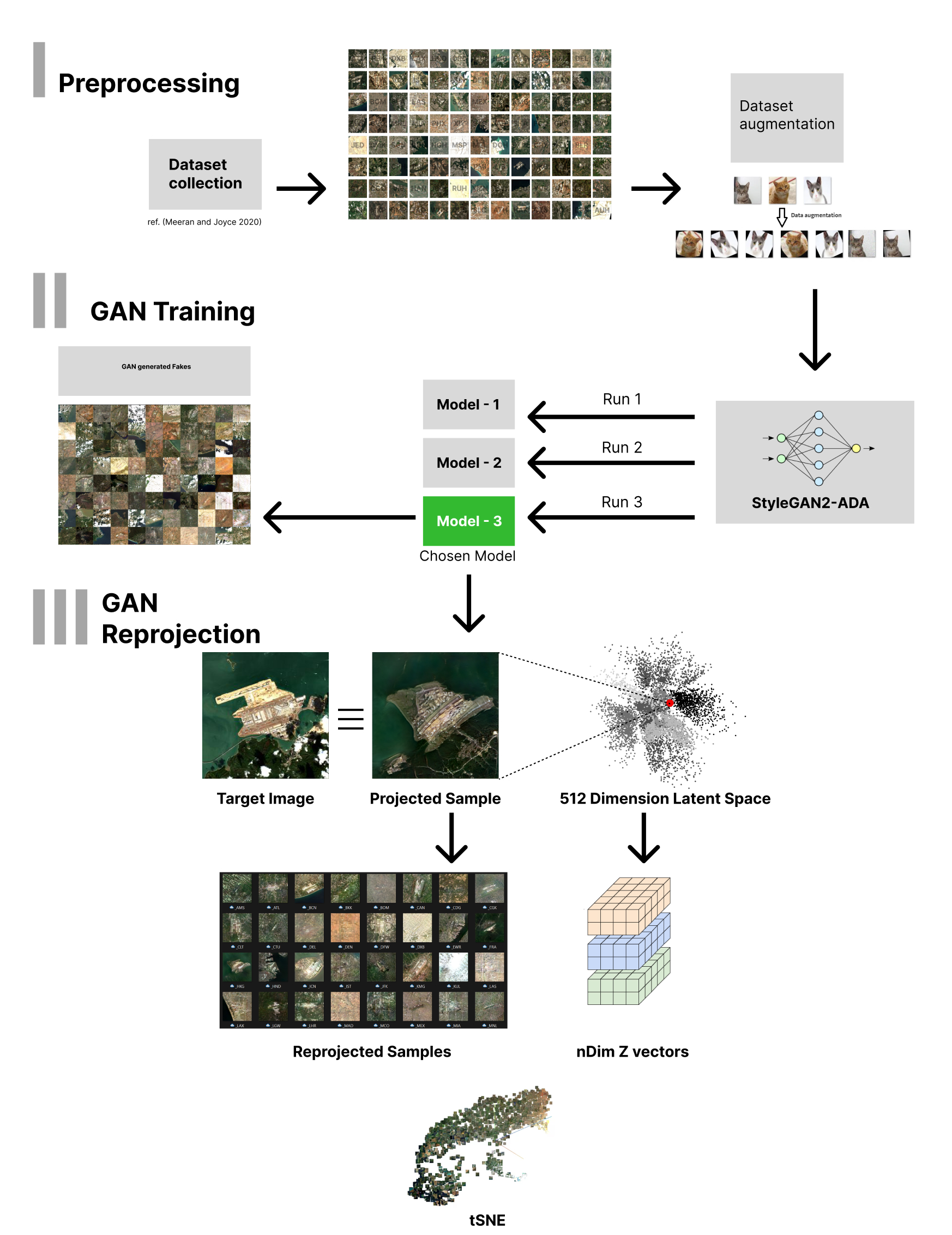
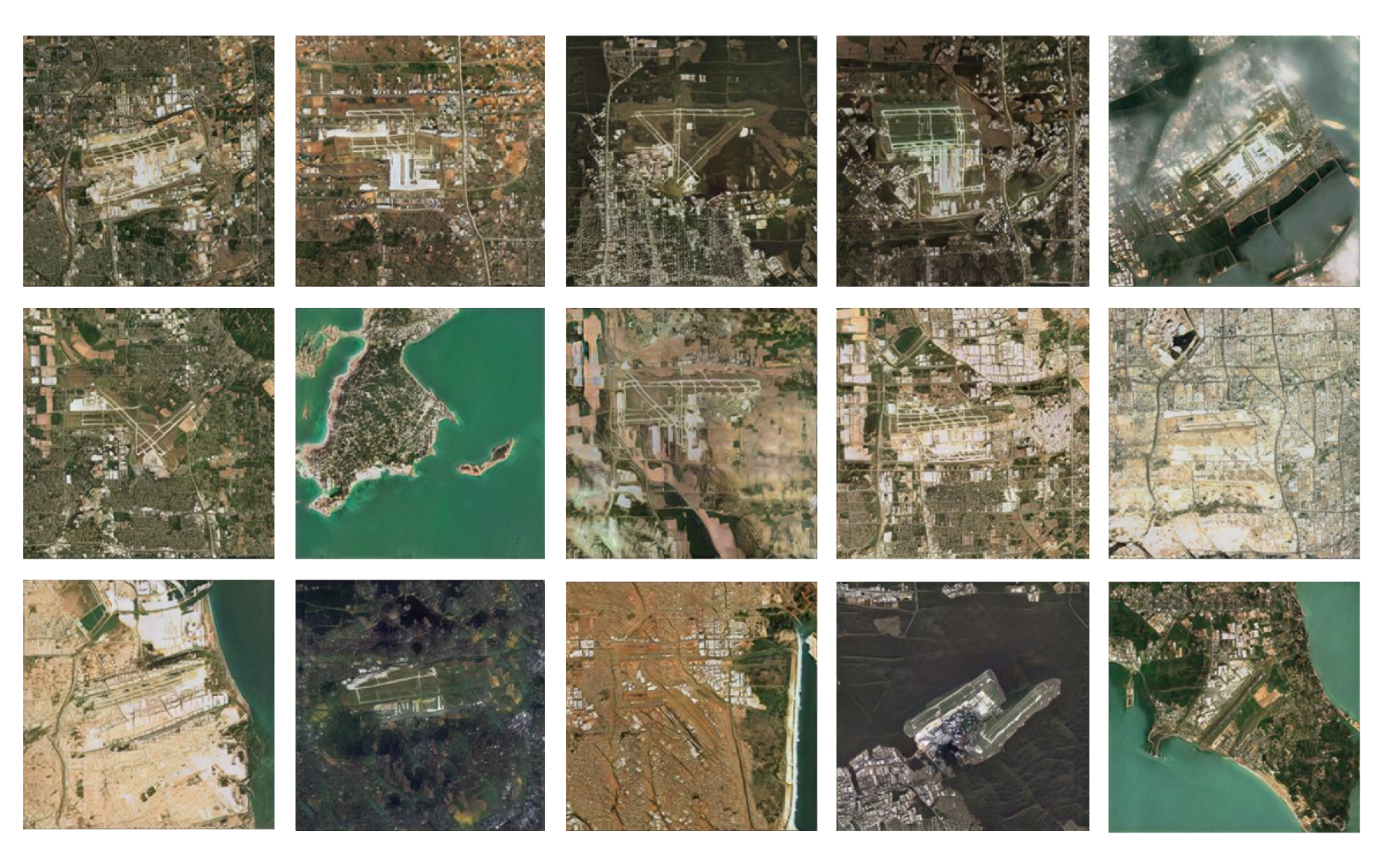
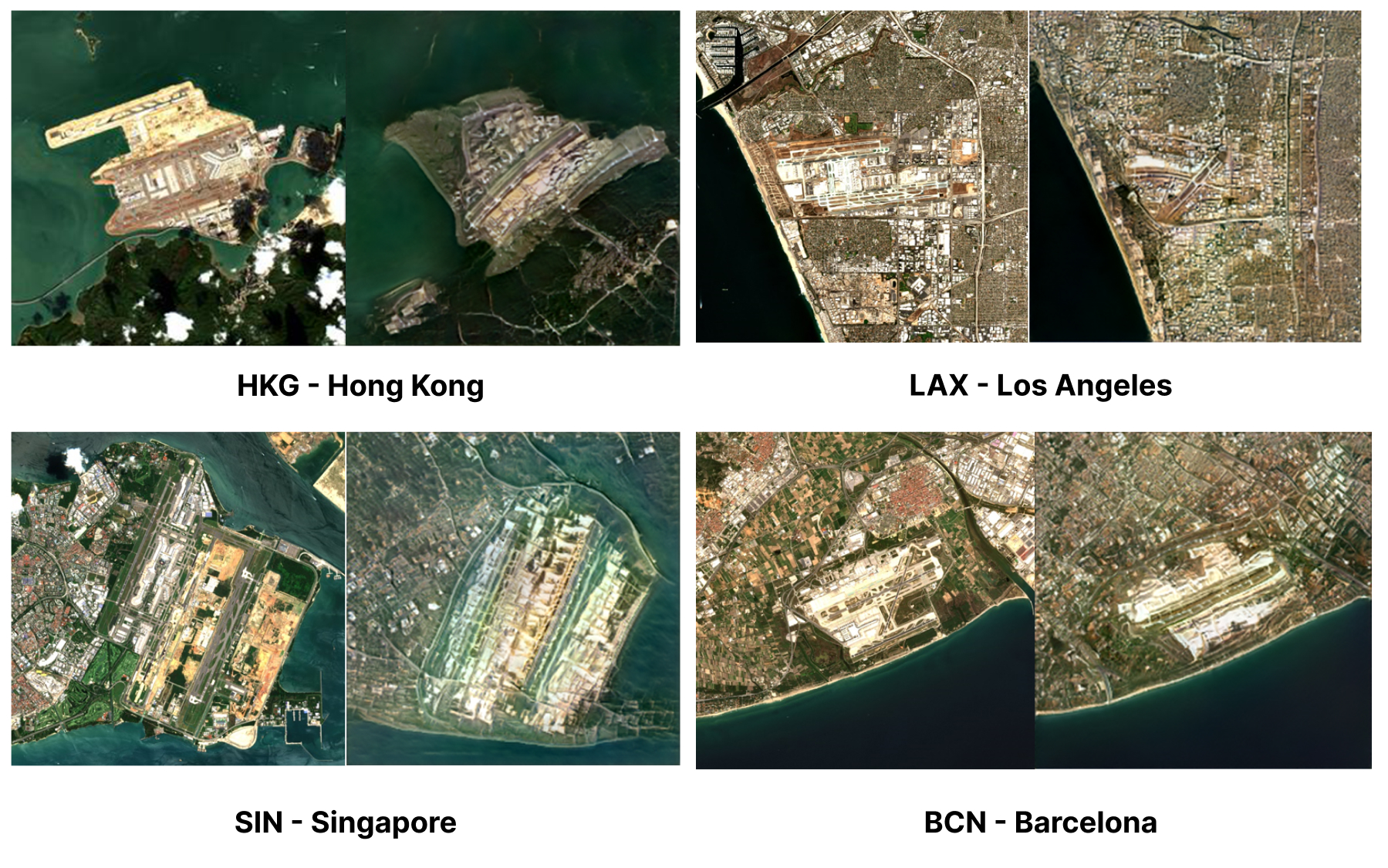
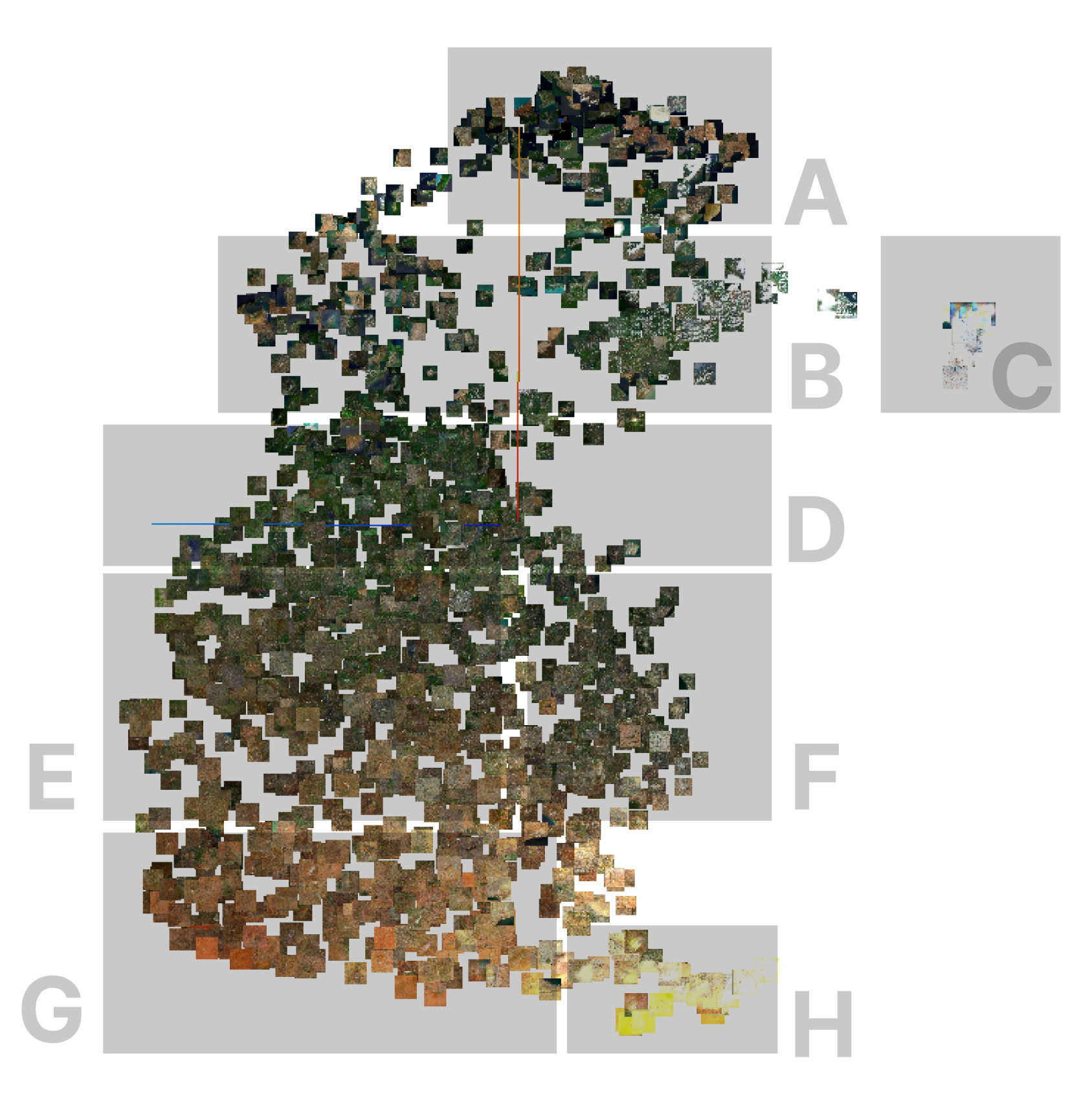
Stay tuned.
If you are interested in this work please get in touch with us.